Franco Caraffi, Marine Systems Director, Costa Corporate I&CT, Costa Crociere S.p.A. and Adrian Schmitt of the data science team at Lufthansa Industry Solutions explain how different industries can learn from each other
THERE HAS BEEN a lot of talk in recent times about what could be done with predictive maintenance analytics. Perhaps that’s not surprising as they can deliver some really interesting outcomes to help users improve the economics of their business. In this article, we want to cover two things with regard to predictive analytics. First, we’ll discuss a little about how to get to a point where you can use the data: what are the problems, the issues arising from this; what are the challenges and methodologies that users have to undertake. The other thing we want to show is what will be next after predictive maintenance. It is likely that some readers will already be aware of prescriptive maintenance but we want to impart a little about that as well.
First of all, however, why are we doing this? Why undertake a journey from data having insight to it having economic strength? And, of course, the aircraft industry is not the only one facing the challenges of maintenance: other sectors such as the cruise ship business have to deal with the same issues. Therefore, we decided to jointly write this article in which we’ll tell you something about Costa Cruises and how what they do is similar to what is done in the aircraft industry; plus we’ll include something about their Neptune platform. We’ll also have a look at Lufthansa Industry Solutions before looking at the project on prescriptive maintenance to show why the industries (Cruise Ships and Aircraft) are similar.
COSTA CRUISES
Part of the Carnival Corporation, Costa Cruises has fifteen ships in service serving guests from 200 countries. To make this work, the business uses 8,300 suppliers on five continents and has 19,000 employees from more than 70 countries. Costa Cruises are sold through more than 38,000 travel agencies worldwide.
LUFTHANSA INDUSTRY SOLUTIONS
As a subsidiary of the Lufthansa Group, Lufthansa Industry Solutions (founded 1997) employs 1300 people today but continues to grow, especially in the area of big data, data analysis, predictive maintenance and the areas associated with that. The group of companies with its head office in Norderstedt, near Hamburg, has several branch offices in Germany, Switzerland and the USA. Besides aircraft MRO, Lufthansa Industry Solutions is also involved with automotive, transport logistics, manufacturing, energy, healthcare and media.
BUILDING A NEW SOLUTION THE FIRST STEPS
A couple of years ago, Franco was requested to take on IT management for Costa Cruises with the goal to create IT surroundings or systems supporting the Captains and the vessels (figure 1).
Figure 1
It was decided that the new infrastructure should take inspiration from the aerospace industry because it was more advanced than the maritime sector… in terms of support, in terms of monitoring and in terms of routing. So, a few years ago, the project started. The goal was to build a system but it was decided to first review the market to see if something was available as Costa is a cruise line not a software house. Unfortunately there was nothing suitable available on the market so the company had to build its own platform to support the Fleet Operations Center (FOC). Since April 2015 the Fleet Operations Center has been working in Hamburg supporting all 26 vessels of the Costa Group businesses. The scope of the FOC is to support the vessels in terms of safety, and marine and nautical requirements. They are supported by the Neptune platform, the tool developed for the Fleet Operations Center.
Figure 2
NEPTUNE; LOOKING FOR TRENDS, IMPROVING QUALITY
Here is where Costa took ideas from aviation; adopting a simple architecture (figure 2) starting from the field device onboard the ships, which sends data, via satellite, to shore-side where that data is displayed on professional nautical charts. Moreover, where the architecture copied that used in aviation IT, Costa also incorporated historical data to be analyzed using the Bridge Operations Quality Assurance (BOQA) system where data could be collected for analysis of deviation and trends, based on which the business can identify policies and guidelines to implement — a virtuous circle that will improve the safety culture and, of course, efficiency.
Starting from safety and safe navigation, we then move to predictive maintenance and energy efficiency using an application originally built just for Costa but later extended to other fleets in the group. Looking to the future, the short video below describes what Neptune does and how it does it: how the data is sourced from the fleet and stored to be used in different applications. It also describes the three areas on which Neptune focuses: nautical safety, protecting the environment and energy management plus displaying information to ensure that each vessel conforms to all applicable regulations at all times. It also covers how the system supports better understanding of the fleet to drive good decision making and continual improvement. And it shows how the system is ready to handle future challenges and leverage future opportunities, such as the Internet of Things (IoT), to improve the planning and execution of maintenance, and the efficiency with which vessels are operated.
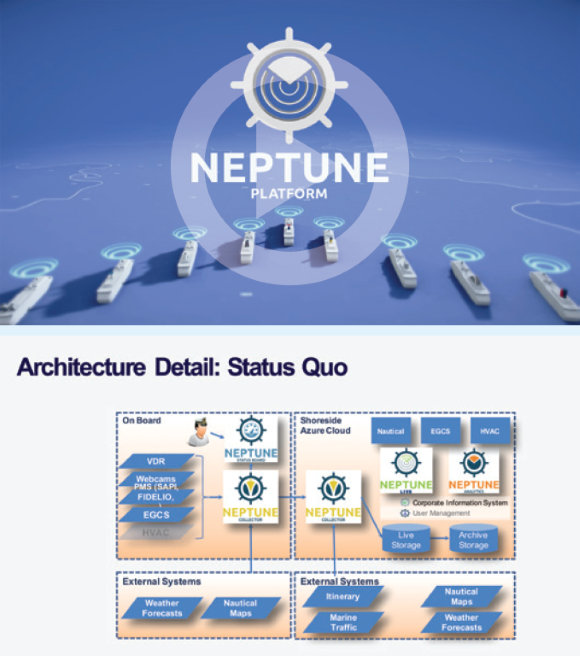
Figure 3
Figure 3 offers an overview of the position today with regards to Neptune and what it does for the Costa Group fleet. It started by looking at engine performance and efficiency, and Costa is now building, with Microsoft technology, a Big Data solution; plus, the business will be downloading roughly 2 million records a day from each vessel. As well as Costa’s own internal needs, the business wants to be able to work with third parties to receive services. The goal is, through IoT, to be able to deliver data to third parties with appropriate expertise who can find, say, patterns for data analytics; and to have this platform available to adopt and apply models for predictive maintenance to improve engine efficiency and energy utilization.
PREDICTIVE MAINTENANCE
Looking now at aircraft maintenance, we already know that predictive maintenance is an important contributor to improving a business but, in the journey to reach predictive maintenance, there are quite a few steps. Also, there is the question of what is being done with all of the results that emerge from a predictive maintenance system. It can be used to improve the business, drive better fuel efficiency and plan more efficient routes; but thinking one step ahead, it might also be a good idea to use insights delivered by the system for one aircraft and connect them with other data to consider how to deal with everything that comes out of predictive maintenance as it predicts faults that will need to be addressed.
FITTING MAINTENANCE INTO THE SCHEDULE
For instance, it’s possible to identify that a component needs to be repaired but predictive maintenance will also note where there are some days of service left in the component so that it might be possible to arrange the aircraft’s schedule to reach a maintenance base as part of its operating roster. With a large fleet, this sort of process will deliver a higher utilization rate and greater efficiency. This is the goal of a project that Lufthansa Industry Solutions is undertaking with Airbus, the DLR (the German Aerospace research center) and the DFKI (the German center for machine learning). The plan is to take all the data from the aircraft and, as a first step, use analytics methods to deliver predictive maintenance, to have advanced analytics in terms of routes and fuel efficiency, and then take this data and project it one step forward (Figure 4).
Figure 4
At the base are the various ATA chapters focusing on the data from one component and from sets of connected components. This data is concentrated and assigned to one aircraft before being used with machine learning to find out how to improve the aircraft; what has to be done next; what is the overhaul, what is the maintenance… However, using the data for an entire fleet means that users can also think of ‘when should this be done?’ ‘where should it be done?’ ‘can it be scheduled with a visit to a base that already has the necessary parts?’ The user has information about the aircraft, about the sensors, about the aircraft’s health and wellbeing plus they have all the other data as well; about where components are located and where are the engineers qualified to check particular components.
TURNING DATA INTO ACCESSIBLE INFORMATION
Lufthansa Industry Solutions is running a project to try to discover all this and has already made good progress with some promising results but the question is; how is this being done? Imagine that there is a lot of data and you want to start undertaking some kind of analysis. The people at Lufthansa Industry Solutions realized that they had a lot of customers who are really interested in this kind of analysis but they don’t necessarily want to build a big system, integrated and using all the data and taking a big step forward. They want to start small and see whether it will work, does it make sense and where does it make sense?
Figure 5
So Lufthansa Industry Solutions helps those users (figure 5) to find and discuss the use cases; then, through small steps, try to analyze them, see the first outcomes. And all the time each step is discussed with the user. Does this help? Is there other data that could also be used to improve those outcomes? Having completed that, it is important to help the user implement the system. Different companies will have various systems running so that introducing another system, training the people to use it and so on is a difficult step. Lufthansa Industry Solutions tries to implement the algorithms, methods and models built for customers in a way that enables them to appear in the dashboards already in use in the business. This is really important to keep people focused and to reduce the time required for a change and the change management needed to change the software.
Figure 6
GETTING THE RIGHT TOOLS FOR THE JOB
Another consideration is what tools will be needed for this? As figure 6 shows, there are a lot of tools available for each step along the data analytics pipeline so Lufthansa Industry Solutions works with customers to identify the best tool by first identifying what are the important things for that customer; and those important things are not only ‘does it have all of the algorithms?’ As we know, most of them are the same algorithms but what matters is how they are set up. It is also really important to see whether these tools have good operational support; is there help for users, is it easy to learn for customers and employees? But, of course, the performance is still very important and how the tool can be used.
If a user goes all the way from having their own data to having their own use cases and, let’s say, puts it in a dashboard or in the right position for them, they need to ask the right questions and need to have good supportive partners.
POINTS TO CONSIDER – SOME EXAMPLES
Here are a few examples from Lufthansa Industry Solutions’ work undertaking this process. What we need to understand is; what are the most important pain points and the most important challenges? We, the authors, came up with three points (figure 7)…
Figure 7
GETTING GOOD DATA INTO THE SYSTEM
The first point is data ingest because, as readers will know, just getting the data doesn’t always mean that it’s correct, on time or using a resilient media for ingest. Of course, with new aircraft, that is not an issue but no fleets will have all new aircraft in the foreseeable future. Therefore it’s important to consider how to get good quality data into the system with a good performance.
GETTING DATA READY TO USE
Second is the data preparation. Lufthansa Industry Solutions has undertaken a number of projects around this and people always think that first you have the data, ingest them and then the analytics come into play. But this is wrong. It is the view of Lufthansa Industry Solutions that 80% to 90% of the time in this process should be spent on data preparation which means checking the data is right and asking does it come directly from the aircraft or are there interruptions where it could be corrupted? This takes a long time and might need to be supported by one or two people in the company whose job is to check this constantly.
THINK BIG BUT START SMALL
Third, if you’re doing this, you need to think big; the purpose is to improve the economic status, how the aircraft operate; that said, it’s important to start with a small step because, if you have a huge goal and can’t achieve it in a certain amount of time, people will get bored, the funding will be questioned and, worst case, the whole analytics and predictive maintenance project might be questioned. Start with small use cases to generate some success stories so that people will see that it’s really valuable. Finally, tools and toolsets are major factors for good data analysis.
CRUISE SHIPS AND AIRCRAFT — SIMILARITY OF NEEDS
We have found that cruise ships and aircraft share the same issues and challenges with data analysis and with predictive maintenance. But we also found some other points of similarity. It’s important to have a realtime health status, to know what’s going on now, not afterwards when an engineer is signing a report. It’s also important to have multi-target optimization in terms of fuel, direction, speed, weather. Third, it’s important to have pattern recognition: this is important because you don’t just want to rely on other people’s conflated experience; you also want to identify further data and insights that you hadn’t even thought about, one of the strongest ties that joins cruise ships and aircraft. Last but not least both sectors need dashboard reporting to show people the outcomes and what they should do.
This, we hope, will have shown readers the similarities of issues and challenges faced by cruise ships and commercial aircraft and how similar data analytics and predictive maintenance solutions can work for both.
Contributor’s Details
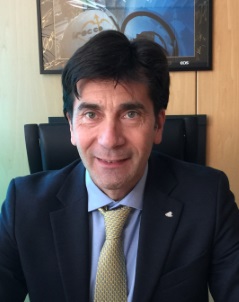
Franco Caraffi
Mr. Caraffi joined Costa Crociere in 1998 and after holding several positions inside the IT organization, he was appointed in 2012 as Marine Systems Director where he has taken a core role in implementing the ‘Safety First’ concept for Costa Group and extending the efficiency model to the safety environment. Having implemented the Neptune platform for the Costa Group, he is now leading the roll-out of the solution across the entire Carnival Corporation.
Adrian Schmitt
Adrian Schmitt graduated in business mathematics (M.Sc.) from the Darmstadt University of Applied Sciences with a focus on data mining and statistics. He was also part of the Competence Center Stochastics & Operations Research (CCSOR) team of the Darmstadt University of Applied Sciences. Adrian Schmitt joined the data science team at Lufthansa Industry Solutions in June 2016.
Costa Crociere
Costa Crociere is an Italian cruise line, based in Genoa, Italy, and belongs to the Carnival Corporation & plc. The Costa Cruises brand currently operates fifteen cruise ships, which all sail under Italian flag.
Lufthansa Industry Solutions
Lufthansa Industry Solutions is a service company for IT consulting and system integration. This wholly-owned subsidiary of Lufthansa Group supports its customers with the digital transformation of their company. Its customer base includes both companies within Lufthansa Group as well as more than 200 companies in various other industries. Lufthansa Industry Solutions emerged from Lufthansa Systems AG on April 1, 2015. The group of companies employs around 1,300 members of staff at several branch offices in Germany, Switzerland and the USA.
To post a comment, please login or subscribe.