Articles
Name | Author |
---|
WHITE PAPER: New Technology Focus – Extending Digital Twins to Digital Financial Twins
Author: Sriram Haran, CEO; Varun Prakash Anbumani, Senior Solution Architect and Vamsi Krishna, Data Scientist, all at KeepFlying
Subscribe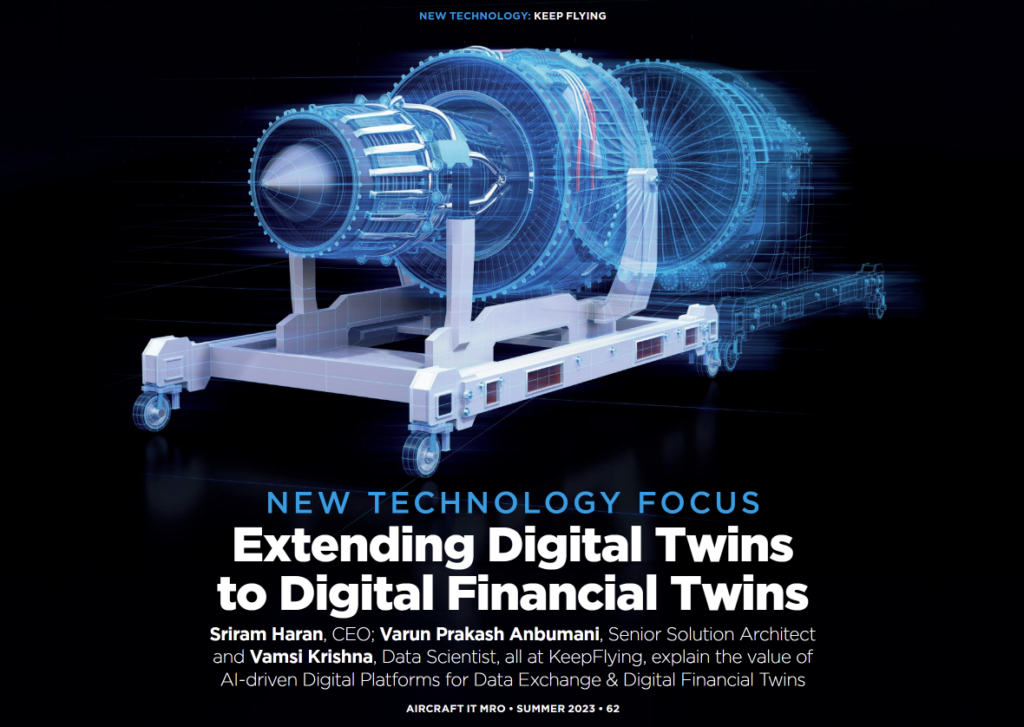
Sriram Haran, CEO; Varun Prakash Anbumani, Senior Solution Architect and Vamsi Krishna, Data Scientist, all at KeepFlying, explain the value of AI driven Digital Platforms for Data Exchange & Digital Financial Twins.
The central theme of this article will be to show readers the case for AI Driven techniques to enhance data integrity and visualize the commercial impact of airworthiness data prior to taking decisions against an Aircraft, Engine or a Maintenance Visit. But let’s start by talking about the importance of data exchange platforms and financial twins in a landscape where profit margins are challenged by Supply Chain and Labor price spikes.
A DIGITAL FINANCIAL TWIN – FINTWIN
There is a lot of focus on predictive maintenance and digital twins these days whereas in reality, during the course of the pandemic, very little was being done to build tools that used the underlying airworthiness and maintenance data of an Aircraft, Engine or Component to build financial / commercial profiles. Our question was: “how can one visualize the commercial impact of a decision being taken against an Aircraft or an Engine before taking it?”. So, we are going to look at a digital financial twin ‘Digital FinTwin®’ (figure 1) which can offer insights with financial implications of decisions.
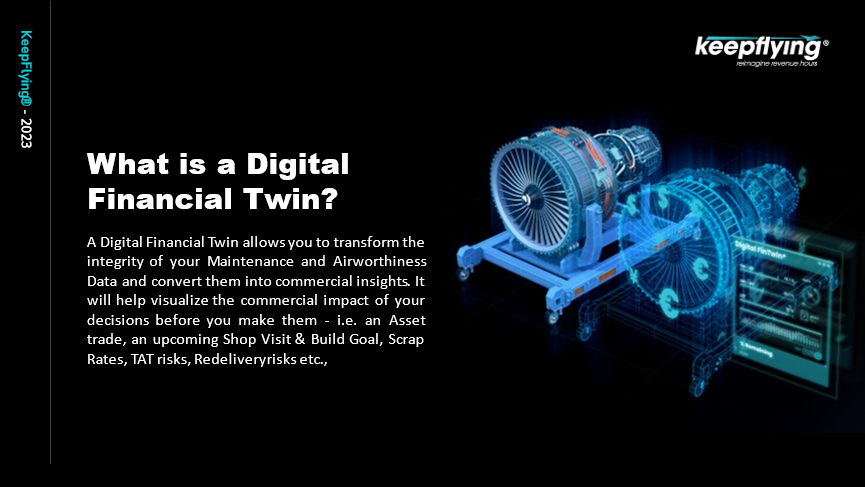
Figure 1
A Digital Financial Twin (Digital FinTwin®) allows you to transform the integrity of your Maintenance and Airworthiness Data and convert them into commercial insights. That then begs the question, what is integrity? For many businesses, data is held in a variety of sources (Excel sheets, scanned PDFs, CMS, MIS | M&E / MRO systems) across departments. So, the first step is to make sense of the data because, only if you can make sense of the data can you apply financial insights on it. That is what a FinTwin® can power. It enables you to understand or realize the commercial impact of decisions before they are made, i.e., an asset trade, an upcoming shop visit, scrap rates (how much will it make, what will be the cost?), TAT risks, redelivery risks etc.
How data science enables the Digital FinTwin®
Let’s start with an opportunity statement focusing on how the industry can achieve Return on Investment (RoI) using such data platforms powered by the FinTwin® (figure 2).
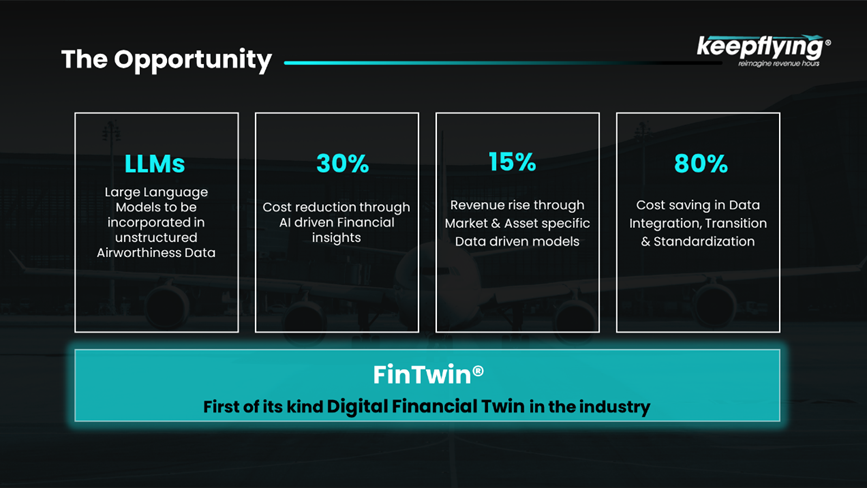
Figure 2
When dealing with unstructured data, it is important that any data model being built is Asset-type specific. This means that the model recognizes the nuances of an A320, or a B737 or a CFM56-5B or a V2500 when creating commercial profiles of each Asset. The fundamental principle to this is what the world is talking about today – Large Language Models. Large Language models tuned to Aviation and Asset specific semantics power the engine that processes large streams of unstructured data that an Aircraft or Engine carries as part of its historic maintenance and airworthiness profiles. This is crucial in achieving accuracy when making predictions around Residual Lives, Redelivery risks, Maintenance Cost Forecasts, Shop Visit Work Scope levels to help visualize commercial impact of decisions around a lease, or a tear down or profitability margins of an incoming Aircraft or Engine maintenance visit.
Paper and metal
The metal has value only when the paperwork supports it: the back-to-birth paperwork related to the metal is very important. That is exactly where a context driven OCR (Optical Character Recognition) is needed to extract only the data that is relevant for the Aircraft or Engine in place. Extraction of this data is followed by a set of rules inherent to the Aircraft or Engine type to check for sanity around LLP lives, applicable ADs, Configuration completeness, statuses of ARCs that are crucial to ascertaining value and mitigating risks during trades.
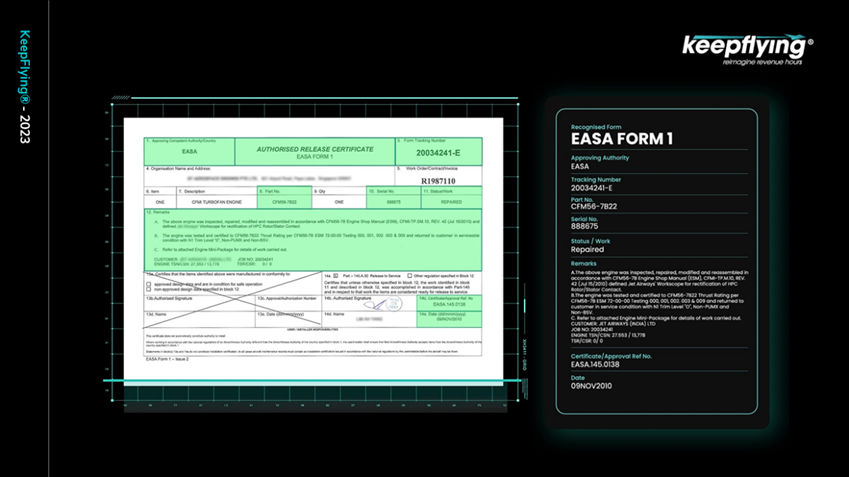
Figure 3
In this image is an example of where data is being extracted from EASA Form 1. Models are trained not only to recognize forms and fields but also to run rules inherent to the Asset type to identify inconsistencies before the data is imported for further analysis.
When the extraction is complete, behavioral profiles are created to continue the commercial impact journey against relevant use cases. For example, against an Engine, trained historic data sets can help complete back-to-birth build gaps by mapping equivalent profiles of Engines that have similar age, utilization profiles, thrust rating, operational environments among other factors.
For an Engine MRO, this can be valuable to predict work scope levels of incoming Engines prior to arrival based on limited or exhaustive data sets. This is crucial in an era of fixed price contracts where NTEP limits can be set and simulated by the FinTwin® to ensure maximum realization of profit margins before slot commitments. The process extends through Gate 0 to save time and effort while inducting an engine and monitors risks and suggests mitigation measures (swaps, exchanges, outsourced repairs, priority swaps through advanced planning and scheduling) to stick to the profitability KPIs.
This is powered through Aviation semantic layers on top of language models that have processed and benchmarked shop visit reports by Engine type and other related parameters (operational, environmental, technical).
This further assists other departments – e.g. Supply Chain – to plan for procurement in case scrap rates are accurately predicted prior to engine arrival. With the supply chain TAT constraints today, this can be a valuable cost saving measure that the FinTwin® can power.
An extension of this use case allows the FinTwin® to help procurement and inventory cost savings through enhanced AOG procurement metrics, smarter stock level management to minimize inventory holding costs and Asset ground times.
CALCULATING REMAINING USEFUL LIFE
An LLP status report typically shows utilization of LLPs across thrust ratings and shows remaining useful life based on cycles limit by LLP and thrust rating minus cycles accumulated. But trained data sets with reliability models can help gauge the impact of environment, thrust rating, derate, EGT margin and other parameters on the failure rate and an accurate calculation of the remaining useful life.
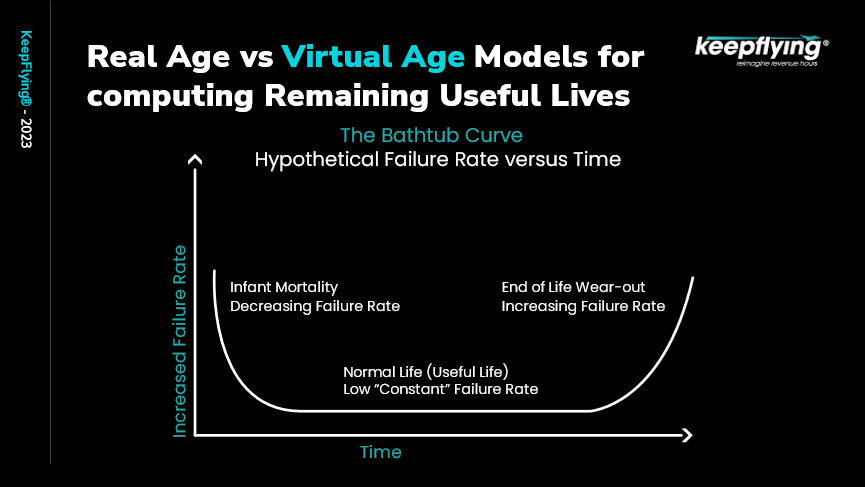
Figure 4
The curved line is the Failure Rate Curve. The start of that curve on the left is for manufacturing defects. There is a threshold for that so when those defects have been fixed and one expects that once you cross that threshold the component will have a normal life. Given that there is wear and tear on that part throughout its usage, the failure rate towards the end of its life exponentially increases because no further repairs are possible. This is what the FinTwin® models using the data which has been extracted.
The FinTwin® computes the cost related to these factors. Factors around macro-economics, inflation rate and interest rates are taken in to account when projecting costs short, medium and long term. KeepFlying® has already published a paper where we have compared deep learning models with the models that we use which can accurately predict the costs and cashflows against Assets. The feature with deep learning models is that they are data hungry, but there are some mathematical models which can resolve this.
Here is an example of one such algorithm, the Dynamic Mode Decomposition, shown below figure 5.
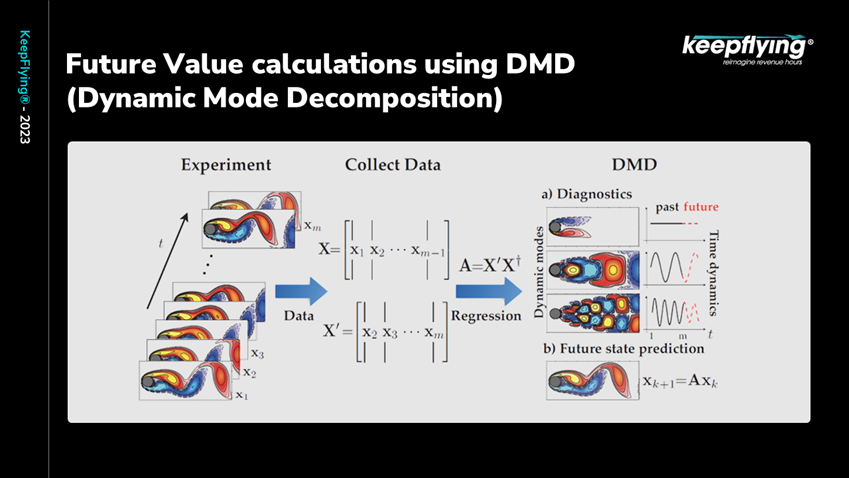
Figure 5
Basically, it is used in fluid dynamics to simulate flow around an airfoil. This can be extended for inflation rates and interest rates, time dependent systems which flow into the future.
All of this is based on historic data and current projections but what about when an anomaly sets in? This is mitigated and improved by a particular aspect known as Bayesian Inference (figure 6).
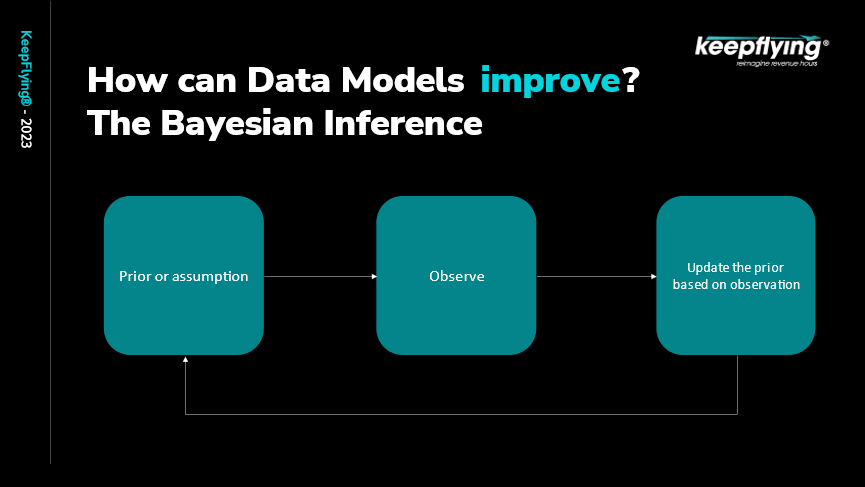
Figure 6
In Bayesian Inference you have a prior assumption, observe what is actually happening and, based on that observation, you update your prior assumption. The main goal is that you take this data and provide the commercial financial impact of trade and maintenance visit decisions. All this is possible because of deep data which is being handled. To handle this data, given the sheer scale at which we are working and the sensitivity of the data we are using, there must be data governance and data privacy aspects to make this whole data modelling in practice.
DATA GOVERNANCE
We know that whatever data we generate will have a dollar value so, when you’re creating terabytes of data, what really matters is the governance of that data to gain full advantage of it. (figure 7).
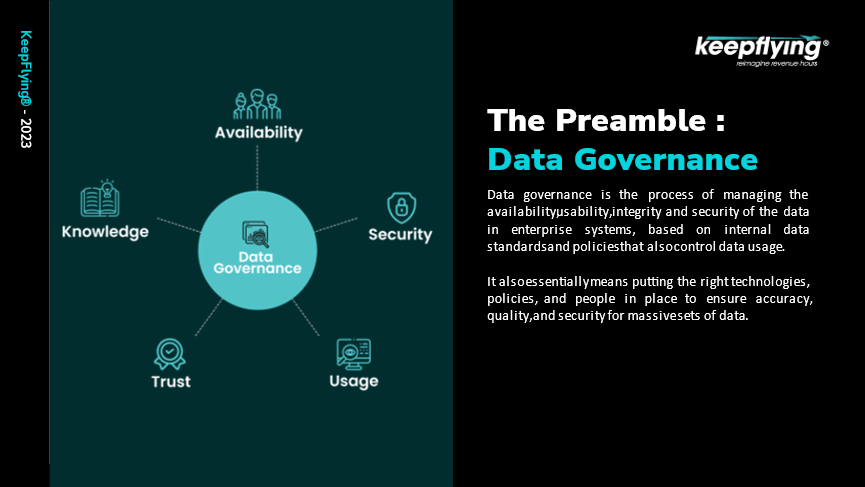
Figure 7
Data governance is the process of making your data available to your consumers in a secure way while maintaining data integrity and privacy. There is a need to create a policy or process which helps you to manage your data efficiently and securely. The reason why data governance is of importance to an organization, especially in the aviation domain, can be seen through these few highlighted problems (figure 8).
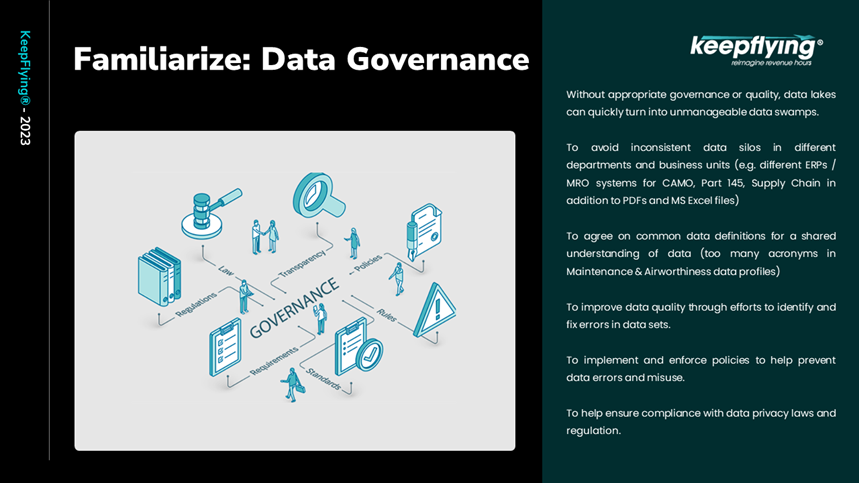
Figure 8
The first problem is that, when you’re creating huge amounts of data and you must store it in a location but don’t have any management tools or anything to organize it: that creates a Data Swamp. The data is just lying there and not being leveraged for any useful purpose. What is needed is a proper policy to manage and use this data and that is one example of where data governance is needed.
The second problem can be that in any organization, data you create in one business unit but when it’s passed to another business unit, they don’t understand that data because there is no shared knowledge of it. That is a problem that many industries face. You need a shared knowledge between business units to be able to share the data vision. If there is no policy, it will be difficult to consume the generated data. This is amplified by the effect that supply chain and labor challenges have in Aircraft & Engine Maintenance profitability.
Another problem is that there are a lot of acronyms in aviation and it’s not possible for a human or a system to understand every acronym. So, a proper definition of those acronyms should be in place.
Each country or region will have compliance and data residency laws associated with it. You need to adhere to those laws. Data governance enables us to be compliant to such laws.
Figure 9 shows results from an interesting survey conducted with multiple organizations and their employees. What came out top are security of data, data quality and data governance.
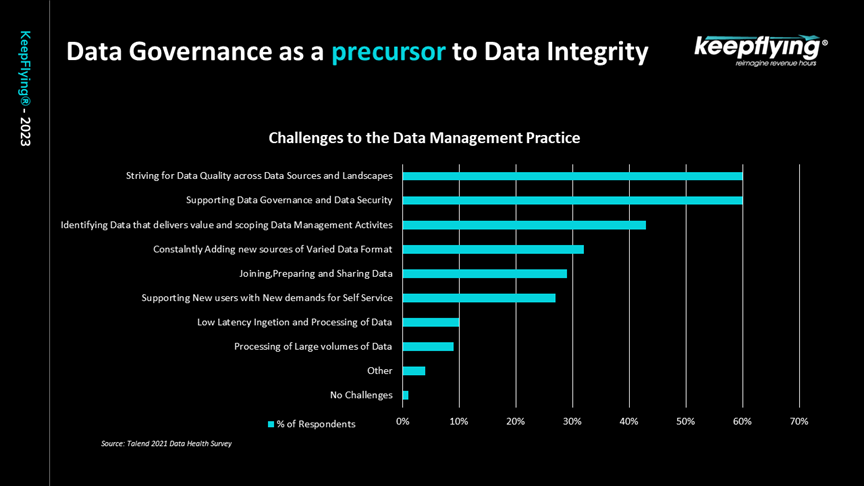
Figure 9
There are multiple ways in which data governance can be implemented but what we are trying to project here is one of the best architectures called Data Lakehouse architecture (figure 10).
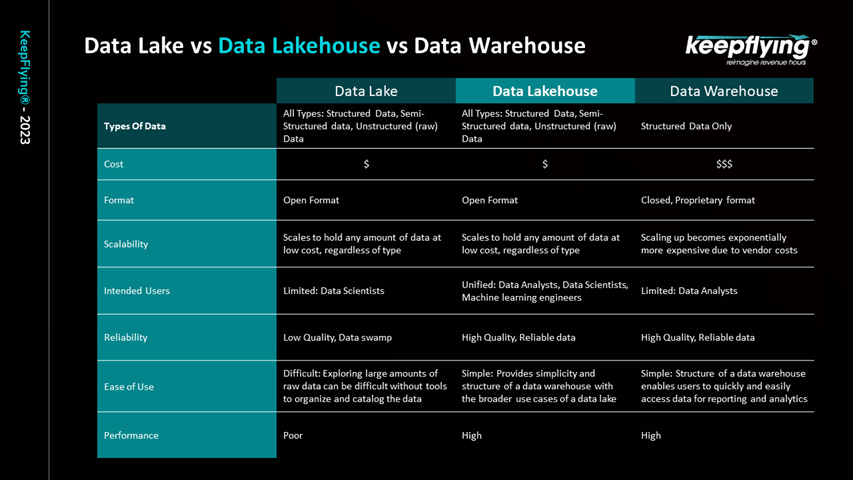
Figure 10
It’s a specification using which data governance can be implemented. But before looking at Data Lakehouse, we need to understand a few traditional architectures. The traditional one is a relationship Database where you can store and work on structured data. It does not have data ready for analytics applications or reporting applications. To solve that issue, the Data Warehouse concept was devised which still stores structured data, but it also supports analytics and reporting solutions with data that can be consumed by them readily. But still there is a problem which is that it can only store structured data, not unstructured data. Unstructured data is when systems generate different types of data and formats all of which have knowledge in it. We cannot just rely on structured data alone. To store structured and unstructured data the Data Lake concept was devised which is a storage system where you can dump all your structured and unstructured data which is then immediately available for data science models and analytics solutions for consumption. The problem with a Data Lake is that, as with a Data Swamp, you can dump all the data in it but there are no tools to manage it.
Considering all of this, a new concept was devised incorporating the best of Data Lake and Data Warehouse. It was named the Data Lakehouse architecture. (figure 11).
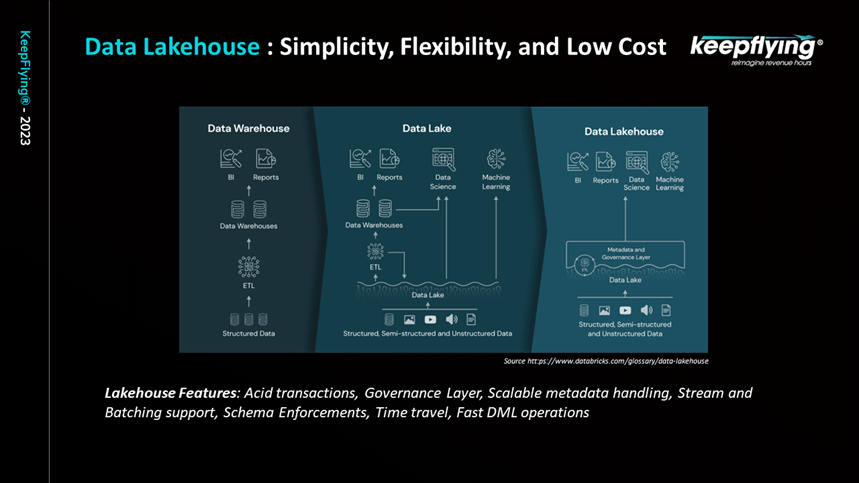
Figure 11
If you’ve worked on a traditional database system, you know that it can provide ACID properties, consistency and more. Similar properties are offered by Data Lake houses. It also helps the streaming and batching of real time data. Systems and algorithms can consistently work on such data. Another important advantage is that a governance layer has been introduced in the Data Lakehouse. It is not present in either a Data Warehouse or a Data Lake. With the Data Lakehouse, you can plug in a governance layer, and, in an automated manner, you can manage your data. Another advantage offered by Data Lakehouse is that it provides scalable metadata handling. For every data in a Data Lakehouse, there will be scalable metadata layers which provide more insights on the data. Any processes that work on such data, such as artificial intelligence or data science models, can work on it and provide meaningful insights. The Data Lakehouse approach is designed to be faster than Data Lake.
Delta lake is one of the implementations of Data Lakehouse architecture which we would like to highlight.
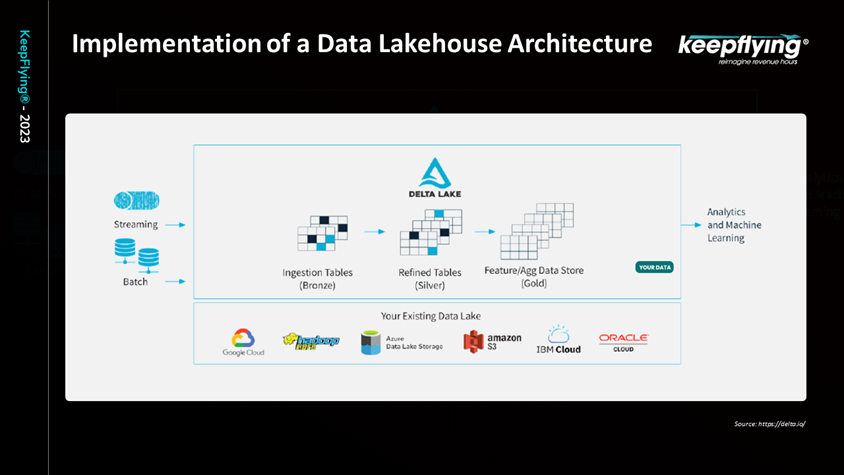
Figure 12
Figure 12 shows all the existing Data Lake systems at the bottom. On the layer above that, Delta Lake has been applied. The data that is streamed into Delta Lake comes out in a well-structured format which various analytics and machine learning applications can use. What you can see in the middle is how the Deta Lake manages data in three tables; bronze, silver and gold. So, whenever data is streamed into the system it goes into the bronze table as raw data with nothing having been done to it. Then transformation and cleanup logics are applied to remove unwanted data, fill out the missing values, etc. and that is when we get the silver table. To understand gold table, let’s consider an example where you have multiple customers and are creating multiple silver tables from each customer’s data. Now, all that is aggregated, and a structure is created that’s called a knowledge structure. The knowledge structure doesn’t know what each customer has put in, it has just gained the knowledge using all customer data tables. Now, we have clean, fulfilled knowledge data ready for consumption and assisting in data governance with good quality data.
The governance layer is provided by some of the tools which can be plugged in to the Delta Lake to achieve a governance layer. Few examples shown in Figure 13. One of the tools is Unity Catalog provided by Databricks which we would like to highlight. It provides a central console where you can look at your data lineage, see what processes are happening plus on your data. It also provides granular level control where you can provide access control at each layer. Governance policies you want to use can be applied on the data using data governance tools. It also assists in reducing Data swamps as we have more insights on data using governance.
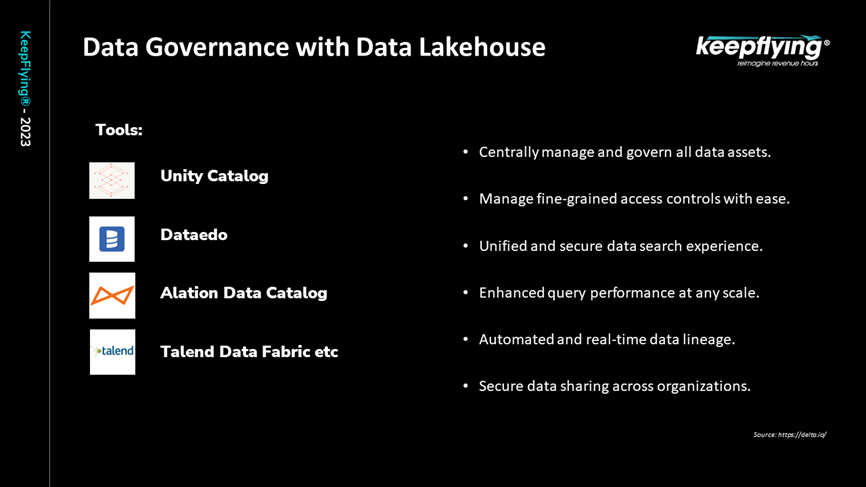
Figure 13
Having covered all this, the most important part is data privacy. We won’t go very deep into data privacy but here are a few ideas on how data privacy can be achieved (figure 14).
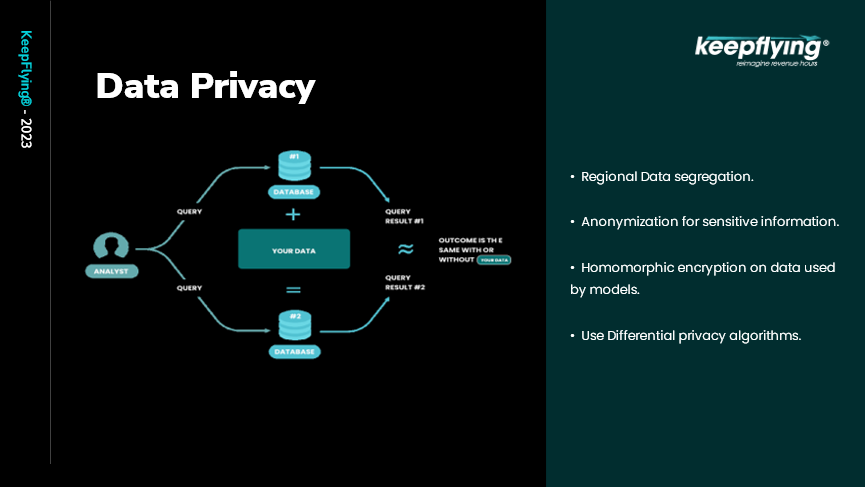
Figure 14
One thing is that, even though there may or may not be any regional data residency laws, you should always try to segregate your customer’s data regionally. It improves performance due to proximity to customer location and also gives confidence to the customer that their data is not shared with other customers. This is one of the simplest ways of achieving data privacy.
Another point is that all your privately identifiable data should be anonymized. One of the approaches called homomorphic encryption can be used for this purpose. It is a concept where algorithms can work on encrypted data without decrypting it which ensures that the algorithms do not know about the personally identifiable data they are working on. This gives confidence to the customer that their data is private.
Another approach growing in popularity is Differential Privacy where noise is purposely added to the data. What this achieves is that, even though we’ve put noise, Differential privacy algorithms ensure that we’re getting the same output as with no noise. It’s like traditional radio systems where, if you tune to a frequency, you’ll hear whatever is available on that frequency but just move the dial a bit and you’ll still be able to interpret the output but with some additional noise. That’s differential privacy.
Data privacy is very relevant to aviation data too. Differential Privacy approach can achieve a good level of data privacy.
We also need to discuss data exchange ecosystems. Aviation is one of those industries where despite technological advancements when it comes to flying, a lot of the maintenance and records keeping processes are still very manual and laborious. Despite efforts by ATA, AWG & IATA to come up with strategies to digitize these processes, we’ve seen various levels of adoption from simple CMS tools all the way until Tier-1 predictive tools like Skywise, Aviatar and GE Predix. Making sense out of unstructured data to help make maintenance and commercial decisions is where AI tools can play a great role in easing the burden of labor heavy functions.
ATA Spec standards (Spec2500, Spec2400 etc.,) are some of the standards which are available to standardize aircraft data. All the data of a particular aircraft can be presented in relevant ATA Spec formats which can be easily consumed by multiple systems. This is one of the ways you can achieve data consistency across an organization.
Having covered the importance of aviation tuned language models, security first data driven platforms, it is down to the sector to make data management arrangements that leverage the most and most useful value out of their data in the most secure ways possible.
Contributor’s
Sriram Haran
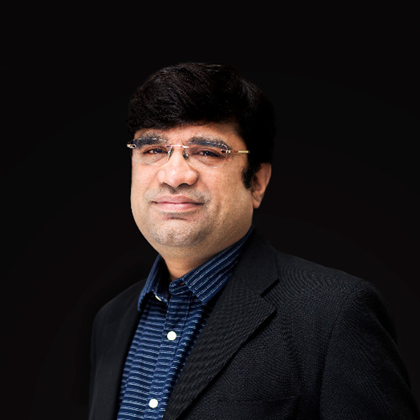
With a strong international trading background, Sriram Haran founded KeepFlying® with aviation professionals. Sriram holds a diploma in Manufacturing Engineering from Nanyang, Singapore and a Bachelors in Computer Science from the UK. He has been an entrepreneur from the age of 21. As Chairman and MD of CBMM Supply Services and Solutions Pte Ltd, Singapore, he has continued to evolve the Group’s portfolio of brands for highly specialized verticals.
Varun Prakash Anbumani
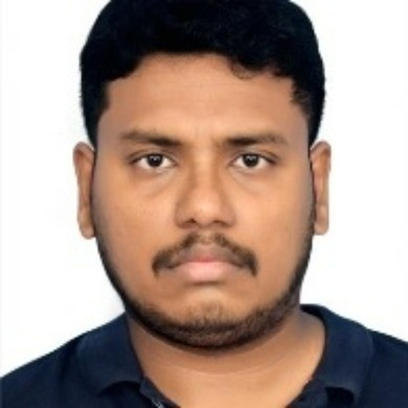
Varun Prakash is a Senior Solution Architect with KeepFlying®. He has Masters in Theoretical Computer Science with 12 years of experience in Enterprise software development majorly in Construction and Engineering domain. He has spent considerable amount of his career working on Cloud Architecture and Cyber Security. He has also been part of compliance initiatives such as FedRAMP and ISO Certifications which are critical for the general processes of a company and security posture of software products.
In his present role at KeepFlying®, he is helping the company with his software development and architecting expertise to launch pioneering cloud-based Data Science products in the aviation technology space.
Vamsi Krishna
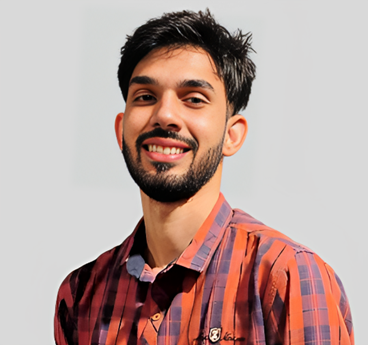
Vamsi Krishna is a Data scientist at KeepFlying®. He has Masters in Computational Engineering and Networking with two Years of experience in building explainable and reliable AI models majorly in the aviation domain. His area of research includes Explainable AI, Dynamical Systems and Data-Driven Modelling. His interests apart from Data Science are in Quantum Computing, Productivity systems, Music Production and Chess.
Comments (0)
There are currently no comments about this article.
To post a comment, please login or subscribe.