Articles
Name | Author | |
---|---|---|
How I See IT: What’s in your solution blueprint | Allan Bachan VP, Managing Director, MRO Operations, ICF. | View article |
Teaching machines to better identify chronic defects | Mark Langley, Chief Technology Officer, ATP CaseBank | View article |
Artificial Intelligence Applications and Advanced Analytics in Aviation Operation | Jahan Alamzad, President, Laminaar Aviation InfoTech Americas, Inc. | View article |
Case Study: Air France – KLM Group – Digitization of Cabin Maintenance | Remco Groeneweg, Project Manager, Air France - KLM Group IT; Michael Lopez, Sales Manager, AirInt Services | View article |
Artificial Intelligence Applications and Advanced Analytics in Aviation Operation
Author: Jahan Alamzad, President, Laminaar Aviation InfoTech Americas, Inc.
Subscribe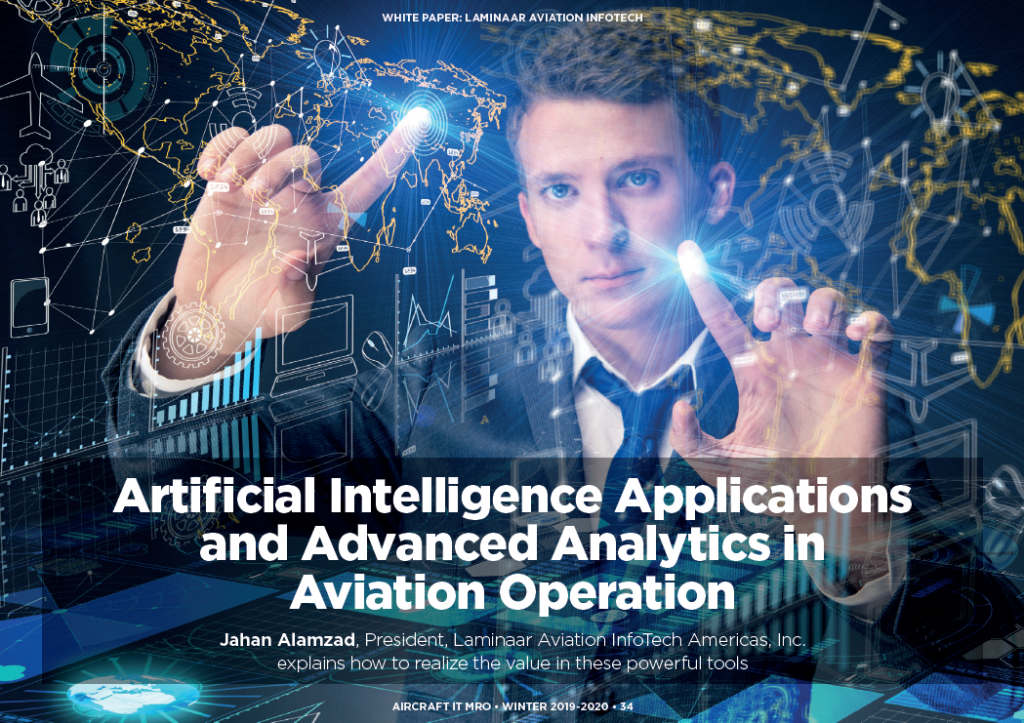
Jahan Alamzad, President, Laminaar Aviation InfoTech Americas, Inc. explains how to realize the value in these powerful tools
Even in recent years, things have moved on when it comes to AI or Artificial Intelligence. We are now mainly talking about applications; beyond the technology as cool stuff, AI has to lead to something and that is application, the theme of this article.
In that context, figure 1 has a specific meaning…
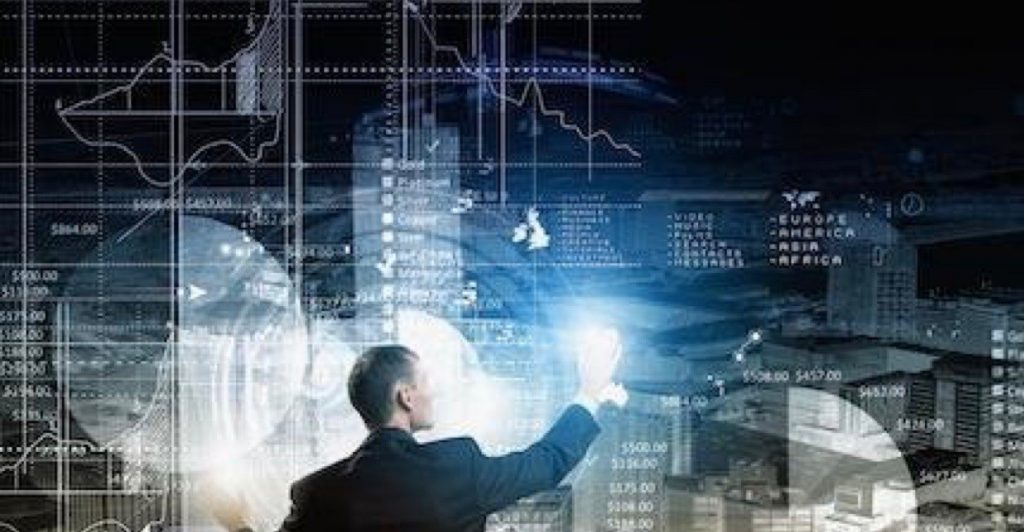
Figure 1
… which should be clear by the end of the article. The article will be divided into five sections with a brief look at the landscape; what kind of innovation technologies can lead into meaningful applications – something where operators can see or experience the benefit in a meaningful and impactful way, that would significantly change the enterprise over the course of time; what kind of an eco-system is needed to deliver those kinds of AI applications and advanced analytics applications; an application development roadmap to get airlines to advanced analytics and AI applications; and then a couple of cases to illustrate how meaningfully these things can come together.
AI LANDSCAPE
The chart in figure 2 includes good news and bad news.
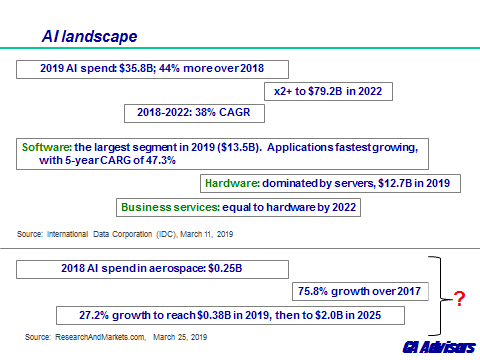
Figure 2
I’m not sure about the accuracy in the lower part of the chart as the sources are different, so there always a danger that things won’t match. The good news is that AI is booming and is going to double by 2022 to the order of $80bn annual spend, the value of all the applications put together, led by software. Software and applications will be a dominant portion of AI and advanced analytics work for the future. Hardware will a close second and, as things stand, business services – implementation, development as a third party provider and support services – are a distant third but, by 2020 will have caught up with hardware.
From this, we can figure out that this will be a significant component of the future economy from the technology perspective. However, unfortunately, this is not led by airlines or aerospace but is mostly by retail and banking services, all the security issues around it like anti-money laundering; those are the primary drivers behind these huge amounts of money that are going to be generated. One item that keeps recurring is predictive maintenance, an item on which all businesses are trying to get a good handle. While I’m not sure about the accuracy of these numbers, the numbers in the lower part of figure 1 represent minimal amounts of that $80bn attributable to aerospace and aviation. The reason for my skepticism is because the global IT spend for airlines is about $24bn annually. We know that it’s going to expand significantly; so if from that, say, $50bn spent, we’re saying only $2bn going to, AI then something doesn’t match.
INNOVATION TECHNOLOGIES
I recently attended a Fintech conference where they, also, were complaining that Fintech is behind as far as AI is concerned and when they talk about AI, it’s really about advanced modelling, machine learning and algorithms, not as we would look at it as purely AI of which advanced analytics are just one component.
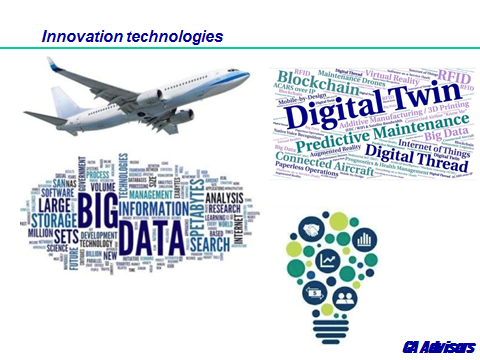
Figure 3
You’ve probably seen the variety in figure 3, talking about different technologies that are used and will be used more vis-à-vis the entire landscape as well as many other exciting technologies like blockchain, which is perfect for airline maintenance operations because it allows users to keep track of the records, guarantee that records cannot be misused or duplicated, altered, etc. Records need to be shown to authorities for e-signature and all the things with which we are familiar and blockchain is made for that task. The difficulty is that until probably last year, people were searching for a general purpose use for blockchain, so there’s something there which can be taken to aerospace and maintenance and that thing will give you blockchain benefits. We are now beyond that. Thinking has evolved. People now look at it as an application on its own, platform agnostic. There is some work to be done, you cannot just buy blockchain off the shelf. The internal development teams at operators need to work with vendors in order to create something that will be impactful for the organization.
AI ECO-SYSTEM
As far as the eco-system is concerned, each of the blocks in figure 4 could contain a lot of words but I’ve removed all words except those that will help us to focus on how the process usually works.
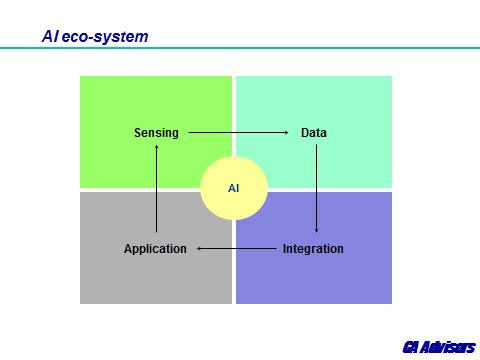
Figure 4
So, any kind of AI that would lead to an application starts with sensing or sensors. Something that is in non-digital format gets digitalized. A company in Palo Alto is working on giving ‘eyes’ to your laptop with in order to look at an object and figure out not only its attributes but what it is. Thinking about that in airline maintenance, for example, you could have a box and let that platform simply see it to know what it is without needing to reference any RFID tag or other identifier; it will know just by looking at it and sensing it. That might take another five or ten years to reach maturity, depending how well the project progresses but those are the sort of sensing things that are happening. In aircraft, all of the ambient information gets digitalized through sensors.
That’s the beginning and then we go into the data piece of it where readers will be very familiar with the term ‘big data’, There are really two components to big data. One kind of data has records and fields with tens of millions of records but the fields do not change; that’s a typical retail example where they need to keep track of millions of items but the fields are the same for each item. With AI, we’re talking about a different type of big data; data coming from different platforms and of different types (weather data, aircraft data, flight plan data…) from different sources. That is important because, once you go into integration and try to build something around that, you need to know exactly where these data are coming from and how they’re going to be used in an integrated fashion. And then it leads to an application, something that can be delivered to a user and that will be impactful.
Where we are now as far as AI is concerned is that a lot of understanding has been built. When the whole AI thing started in the late 1960s, the idea was to come to human level intelligence. However, the emphasis is now different with a focus on AI helping users to make decisions better; helping to do some kinds of physical activities. That’s the big difference.
The structure of artificial intelligence is amorphous; each user will have their own understanding of it but reaching to a human level fully cognitive state is not currently on the cards. An example of fully cognitive would be that I recently made a reservation for lunch in Palo Alto and then I needed to make another reservation for the next day at the same restaurant. When I arrived on the second day, the lady who was greeting customers said ‘but you were here yesterday’. She had gone through a cognitive process and concluded that, just because I was there yesterday, I probably shouldn’t be there the next day. This sort of cognitive process is very difficult to program, to code; it is very difficult to get to that stage. However, you can program a robot to, whoever shows up, just say ‘hello’ and ‘that’s your table’; we can do that. Some might ask whether that is scary and it is if, when we achieve fully cognitive AI, we misuse it; but the good news is that we won’t be getting there any time soon.
One benefit is that AI can be optimized to maximize turnaround and repeatedly do the same thing with accuracy and reliability. Again, many might ask whether that is going to replace the human touch but I don’t think that will happen: rather, it will create another sort of work, the flavor of work changes so we will all benefit.
AI AND ADVANCED ANALYTICS
Coming to advanced analytics, that has a concrete structure, we know what it is, we know specific models that we are using to deliver an application.
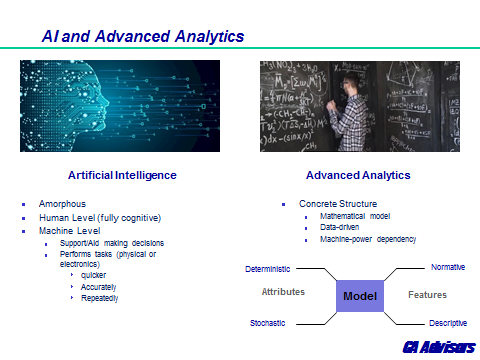
Figure 5
Typically, we divide that into either normative or descriptive models (figure 5): normative are the ones that instruct, ‘that’s the best solution’; descriptive is a typical simulation model, you give it a situation, you simulate it, you get the attributes of that ‘good or bad’ but it does not return with a value judgement on that process.
Or it could be deterministic or stochastic. Linear programming is deterministic, there’s no probability attached; a crew is either on a flight, or not, or dead heading. It’s the same for network optimization; an aircraft type covers one segment and it does that, there’s really no probability of an aircraft covering one flight. Stochastic models have probability included like simulation modelling.
Particularly for maintenance processes, if you go to any engine shop, for example, the engine comes in and really the processes are not set, there is not a plan, until the engine has been opened, the inspector has looked at it and then directs what to do, which work stations it should go to. That is significantly different from manufacture. In manufacturing, there is a pre-ordained production plan, but MRO is a much harder problem than typical manufacturing and that is where AI comes in. But, if it’s such a difficult problem, why are we not investing more in it as compared to, say manufacturing where AI gets a big share of investment?
APPLICATION DELIVERY ROADMAP
As far as a roadmap is concerned the bubbles in figure 6 have specific meanings and represent gateways that start from one point and end at another point.
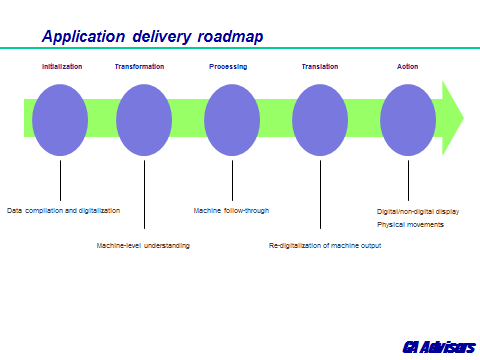
Figure 7
The reason that this breakdown should make sense is that there are different vendors offering different portions of this value chain or this road map and there are different resources allocated to each part of it. That’s why we are way beyond the original thinking that one needs a general purpose platform of AI to bring in, morph into your needs and that becomes the application. That is no longer the case: today we need to be very specific about our approach; you could not get a general purpose AI and try to build applications around it.
It starts with getting the data together before launching into developing and applications. For many readers who are familiar with regulatory authorities and the way that documents are issued for operators to comply with, the first step is data or information; you get everything you need first before doing anything beyond that. As it is with analytics and AI, you first need to put together the information. You do not want to develop an application that, in the middle of the process, needs more information, that’s not the way to develop an application.
Then there is the translation of whatever has been put together into machine understanding. There are different vendors working on making that much easier. When the machine starts the process, it doesn’t look at the generic codes the user puts in, it needs that kind of a translation. What makes AI and particularly Advanced Analytics faster is that these gateways need to be seamless. For example, I just tried to change my airline seat and was waiting for almost one minute for my record to appear and the reason for that is just because of the different gateways that it goes through to get the reservation records from the database as you do not touch the database directly, so it has to go into a mimic, a mirror image of the reservation system, for one record: and then it goes through the GDS and then I’ll see it. And since there are different platforms involved, none of which were synchronized during the development, it takes a while for the record to show up. In AI that is real time applications and in order to avoid that type of delay, these gateways need to be very well thought through and the data hand-off to work very smoothly.
CASE: PREDICTIVE MAINTENANCE
Predictive maintenance (figure 8) is really not just one application that you can point to; it’s a combination of AI and Advanced Analytics to begin with. But there are three segments to predictive maintenance.
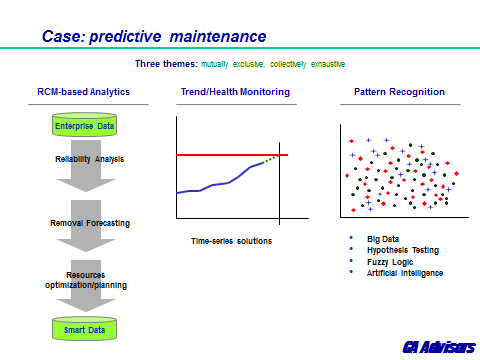
Figure 8
One is planning, reliability centered, maintenance (RCM)-based applications. That’s regulatory mandated. And based on that, applications are developed in order to find out certain things. For example, we cannot say which engine on which aircraft will fail at what time, that’s not the best use of AI. But we can say, ‘based on what data I have, we can say what the probability is of those events happening’. With ten engines flying and each of them having a ten percent chance of failure, it’s pretty safe to say that there will be one engine failure; we don’t know which one or at what time but during the time period covered, we should plan for one engine removal. So that’s on the RCM-based planning side.
Readers will be familiar with Trend/Health monitoring. Condition monitoring, real-time monitoring has been with us since RCM was introduced and MSG-3 (Maintenance Steering Group) mandated that, for things like aircraft engines and other systems. We can develop analytics that will predict when something will happen and, again, there are tools available now that can do that. So, we can look at the condition of engines, look at degradation and then say, within the next two months, something bad might happen. The problem is that in all that modelling, that trend might go up but then levels off for a long time. So we predicted this engine to fail within two months but seven months have passed and nothing has happened. There are caveats when dealing with this sort of time-series trending. There are different types of exponential smoothing that can be done but, in general, we cannot expect 100% accuracy.
The third theme is pattern recognition and, again, there’s a lot of money going into that, particularly for life sciences, trying to find the patterns in very large quantities of data and whether that’s something that can benefit your organization. Again, we are not there but we’re at the beginning of pattern recognition.
You hear different things like, whenever the cargo door opens up, some fuse on the other side of the aircraft burns out but does that happen all the time? It is around those sorts of pattern recognitions that people are trying to build applications.
CASE: DAY OF OPERATION RECOVERY/REPAIR
Figure 9 illustrates what is really the latest position that people are talking about for applying AI and Advanced Analytics for disruption management.
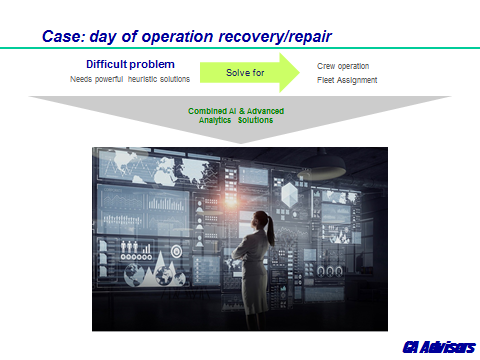
Figure 9
When something bad happens, like weather over an airline’s hub, it is hard to recover from that. A lot of operators and a lot of vendors are working on it but, as yet, with nothing applicable emerging. The difficulty is the magnitude of problem. To give you some idea; at the moment, the largest mathematical programming optimization that can be solved by the most powerful solver consists of 115 million variables with 40 million constraints and takes about 2 hours with a regular 365 powered machine. If you talk about crew management, for example, with 250 to 300 aircraft types, that takes about 4 hours to reach optimization; that’s the planning side, which crew covers which flight: on the disruption side of it, that is ‘that crew needed to be on that aircraft but it’s over there’; if you want to solve it for today, maybe the next two flights, that’s more than double that amount, so it takes about 5 to 6 hours to solve that. And you don’t always have that luxury; you usually need to make a decision faster than that.
So, again, we are not yet there. What we have are just steps towards that which requires a very powerful heuristic algorithm and they are typically highly guarded, nobody discloses them. The typical developer is developing strategies and then developing mitigating heuristics for that heuristics algorithm which they put together for the entire day. It’s not an optimal solution, it can improve but, at that time, when you are trying to recover from disruption that’s really the best we can do. Just the recovery side of it and the turnaround is still a major task. Without having any kind of a tool, it will be quite disruptive for the entire day for that to happen.
Contributor’s Details
Jahan Alamzad Biography
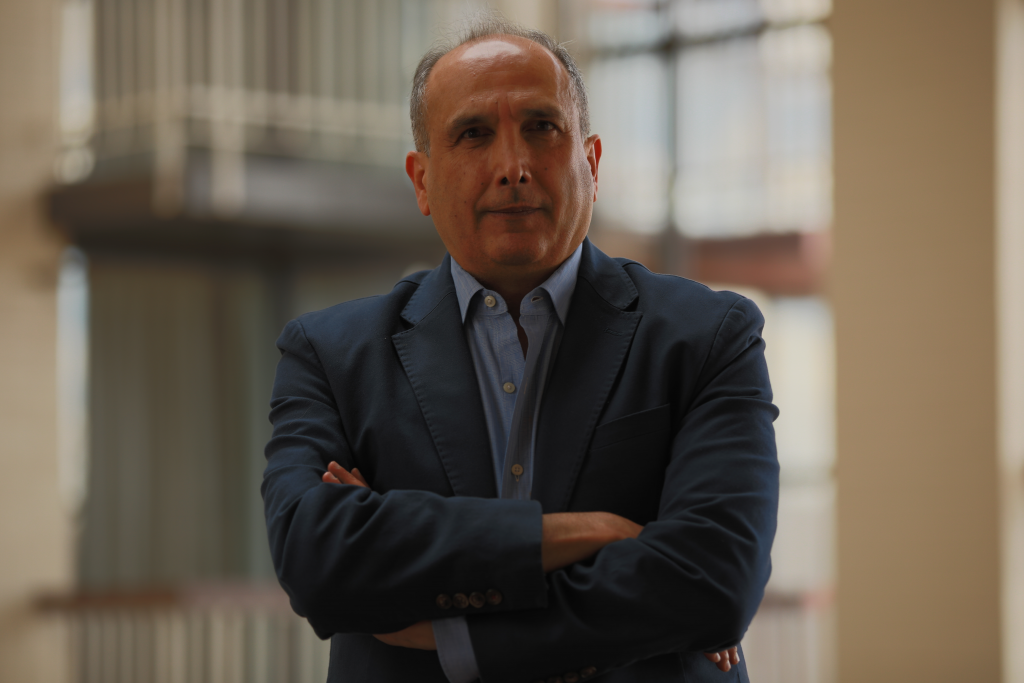
Jahan Alamzad previously served as managing principal of CA Advisors. He was also director of strategy and analytics at PricewaterhouseCoopers, and director of airline, aerospace, and operations research at Applied Decision Analysis, Inc. He has worked at American Airlines and United Airlines, co-founded California Airlines and was its CEO. He has served on the faculty of the School of Business and Management at Notre Dame de Namur University, and on the faculty of the Department of Aviation at San Jose State University. Jahan holds a masters in management science and engineering from Stanford University.
Laminaar

Laminaar offers a future-ready and fully integrated software applications suite for the aviation business: airlines, non-scheduled operators, MROs, airport operators, regulators and training facilities, with in-built optimizers, business intelligence (BI) & Data Analytics. Solutions may either be accessed as a complete suite covering the full spectrum of operations, or as a stand-alone module addressing a specific functional area. The suite has a unified database that allows a seamless flow of data and information between operational functions.
Comments (0)
There are currently no comments about this article.
To post a comment, please login or subscribe.