Articles
Name | Author | |
---|---|---|
Using big data to schedule unplanned maintenance… streamlining the A&D support chain | Brendan Viggers, Product & Sales Support, IFS A&D Centre of Excellence | View article |
Column: How I see IT – 3D, or not 3D? That is the question | Paul Saunders, Solution Manager, Flatirons Solutions | View article |
The Internet of Flying Things: Part 2 | Michael Wm. Denis, a renowned author, speaker and independent consultant | View article |
‘Less is more’: MRO Software Implementation at FL Technics | Ramunas Paskevicius, Head of IT Unit, FL Technics | View article |
Case Study: Rotary Wing MRO IT Case Study | Jan Ketelsen, Manager Maintenance Planning, ADAC Luftfahrt Technik GmbH | View article |
The Internet of Flying Things: Part 2
Author: Michael Wm. Denis, a renowned author, speaker and independent consultant
SubscribeThe Internet of Flying Things Part 2
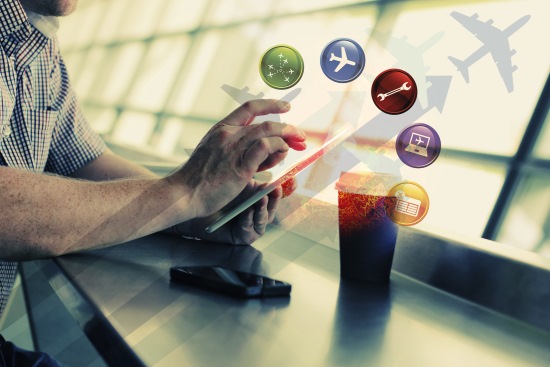
In this second of two articles, Michael Wm. Denis presents valuable considerations for Internet of Things initiatives and how companies can profitably cash the check of predictive maintenance
Walking aboard Delta’s new A350-900 XWB, you are impressed with its lighting, 1-2-1 herringbone layout and, of course, the plush lie-flat seats. It definitely appears your flight to FRA and onward to the Aircraft Commerce MRO/OPS IT 2020 conference in Darmstadt will be quite pleasurable. Snuggling into the wide birth and pitch of the Zodiac BusinessElite seats, the amenities are immediately apparent, starting with the Thales IFE, USB ports, power chargers and GoGo Ku-band satellite Wi-Fi that covers the entire international flight.
As the aircraft revs its engines at the end of the runway and then shudders slightly when the pilot releases the brakes, you momentarily flash back five years, remembering the engine fire on BA 2276 at LAS McCarran International Airport. And it was during another aviation maintenance conference that you sat next to the Qantas Director of Maintenance (DOM) as his mobile phone kept ringing during your joint presentation, only to later find out that A380 QF32 had suffered an in-flight uncontained engine failure and was limping back to SIN Singapore Changi Airport.
But today’s flight will be uneventful, knowing that Delta had implemented predictive maintenance, dynamic diagnostics, prognostics and health management systems in 2016 and such events, as rare as they were before, had become non-existent. After a wonderful meal, wine and movie, you pop out your laptop to put the finishing touches on your presentation, The Internet of Flying Things – Lessons Learned Five Years Later.
While safety of flight and reliability are always key success factors within the aviation community, it was financial return that motivated most airlines and OEMs to invest in predictive maintenance and prognostic health management. William T. Greene, Vice President, Technical Operations Finance and Strategic Planning at American Airlines, always used to ask a simple question when someone brought him a bright idea, “How do I cash that check?”
The Internet of Flying Things, that is how.
Predictive maintenance
This article picks up from where part 1 left off; understanding what the IoT is, how do we go about realizing the potential and turning it into real value.
There are plenty of everyday manifestations of the IoT around us already. A SmartHealth watch senses our vital signs, interprets our sleeping pattern and current health in order to determine or prescribe when we should wake up, decides which of a plethora of home appliances to turn on based upon one’s personal schedule, and then responds by communicating to and controlling a SmartAlarm, SmartLighting, SmartHVAC, and maybe a SmartToaster. Of course, it might be apposite to ask, do we really need a ‘Smart Toaster?’
In the Google Nest example (see previous article), their SmartHVAC doesn’t merely provide remote control and static remote programing of temperature set at specific times. Via geolocation sensing, Nest knows when people come and go at the house, what the weather is at the home’s specific location now and in the future and over time, via machine learning, develops personalized behavioral patterns. This gives Nest the ability to optimize a home’s temperature and humidity specific to the user and specific to the constantly changing environment. With the addition of smart remote control dampers people can make use of Modern HVAC installed rooms as it can easily reduce energy costs by directing heat or cool air to specific rooms that residents are actually in while preparing other rooms for future use, say a bedroom just in time for sleep.
Nearer to home for MRO is predictive maintenance (PdM) and it’s much more than predicting component failure. PdM builds upon RCM (reliability centered maintenance), CBM (condition based maintenance) and an operator’s current MSG-3 maintenance program from the ATA’s maintenance steering group – tailored for each specific tail or rotable. PdM is a cyclical group of capabilities that enables continuous improvement in reliability, quality, safety, asset performance and total cost of operations.
Predictive maintenance augments traditional maintenance methodologies (RCM, CBM…) and the capabilities and processes that everyone performs today. What is fundamentally different is predictive maintenance gives us time to actionably act.
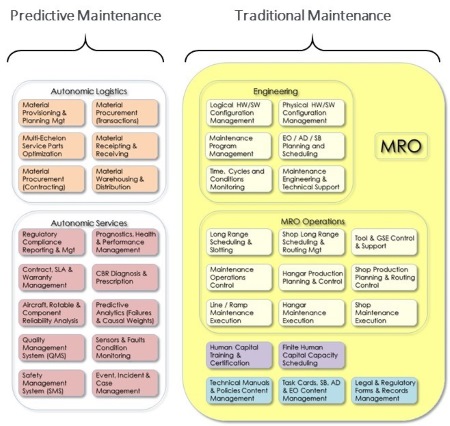
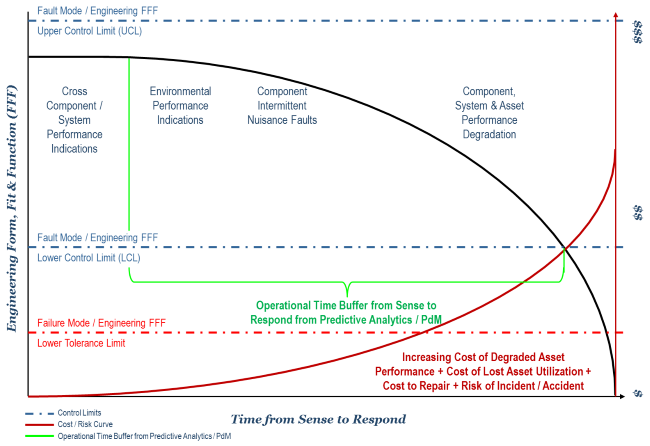
The first of these is pretty obvious. A sensor or inspection indicates at least one critical engineering attribute is outside of a tolerance level and it truly is. The converse, non-failure, is when a sensor or inspection indicates an out of tolerance condition but it actually is not. This is called a false positive or Type I error. There is also what is known as a false negative or a Type II error.
So prediction identifies that a component is headed down its RCM P-F (interval between Potential failure and Functional failure) curve at some point in time before failure, given some level of predictive accuracy or it predicts and prevents no fault found. The bottom line value proposition of prediction is delivered in time. In the diagram, it is the time to respond prior to failure or non-failure without taking a revenue generating asset out of revenue generating service.
Dynamic diagnostics.
Traditionally, diagnostics has been considered a lagging or reactive capability – that is – engineering form, fit or function is already degraded, fault mode codes are being sensed or full failure has occurred. While in many cases this maturity level of diagnostics is still commonplace, especially when using static TSM (troubleshooting manual) or FIM (fault isolation manual) manuals, in the context of predictive maintenance and predictive analytics, diagnostics is becoming proactive. Customizable diagnostic reasoning engines that operate on a database of known equipment issues (i.e. a fault isolation) can capture all of the symptoms, causes and solutions for every known failure mode for a specific type, model, series or even individual piece of equipment. By dynamically generating decision logic, these proactive diagnostic systems identify the root causes of problems to guide users to the right prescriptive and corrective actions.
Jeff Immelt, CEO at General Electric succinctly sums up the new world order, “If you went to bed last night as an industrial company, you’re going to wake up this morning as a software and analytics company. The notion that there’s a huge separation between the industrial world and the world of digitization, analytics and software is over.”
And Mr. Immelt’s observation is at the center of much of the increasing complexity in diagnosing faults in aircraft systems and components, the constantly changing hardware, software configurations and the static nature of paper or even electronic content management systems.
At the April MRO and OPS IT conference in Miami, Airbus presented their long range plan to incorporate predictive maintenance and advanced diagnostics capabilities into their OEM (original equipment manufacturer) specific solutions and we can be assured that Boeing, Bombardier, Embraer, GE, Pratt & Whitney and others will also build OEM asset specific tools. But even if an airline is a single fleet operator, unless they also perform all of their maintenance, repair and overhauls in house – I don’t know any who do – then OEM specific tools are no real enterprise solution. And of course the OEM-MRO ecosystem requires open standard interoperability across Information and Operations technologies and architecture for the entire industry to realize the value of everyone’s investments, something that mere data standards will not solve.
Predictive maintenance must be implemented in actual airlines’ current and future technology systems. IoT is commonly described as combining Information Technology (IT) and Operational Technology (OT). IT is exemplified by ERP (enterprise resource planning), SCM (supply chain management), CMS and MRO systems whereas OT includes the on board Central Maintenance Computer (CMC), engine control and avionics systems and even near board systems like diagnostics or health management systems. An aviation OT architecture might appear like the one below:
To actually realize value from PdM capabilities, IT and OT systems will need to be integrated and matured. Doing that starts with an understanding of the state of these systems today and a plan on where to go moving forward. And because IoT is an ecosystem set of capabilities, they cannot be integrated or matured in a vacuum. If your aircraft or engine or component OEMs don’t enable OT sensing or if your MRO vendors don’t improve their MRO, CMS, digital and mobility capabilities, then building the best internal IT and OT will mean realizing less value from your investment thus increasing the costs for everyone in the aviation ecosystem.
Servitization: performance based business models
Probably the most disruptive element of IoT is how it enables servitization of ‘products’ or ‘devices’ and thus totally changes a company’s business models. It is interesting to note that, in the general media, the most often cited example of how IoT fundamentally changes a company’s business model via servitization is Rolls-Royce. We in aviation are very familiar with ‘Power by the Hour’ and how it bundles a ‘product’ or ‘device’ funding, purchase price, warranty, maintenance, service parts, etc… and denominates the price paid in what is actually being consumed – thrust. This is what is meant by ‘servitizing’ a ‘product’.
In IT this is commonly referred to as Software-as-a-Service (SaaS), Infrastructure-as-a-Service (IaaS) and Platform-as-a-Service (PaaS). Salesforce is a prime example of both a PaaS, where any software vendor can develop and host their solution, while also being a SaaS for their own CRM solution. And in order to optimize revenue and profits and market share, Salesforce PaaS hosts competitor’s CRM SaaS solutions. This is what is known as coopetition or the combination of cooperation and competition.
The greatest challenges for companies from this point on are not technological – transport protocols, data standards, security, connectivity, etc.; rather, they are centered on realigning their thinking, strategy and business models for servitization, a future that has already arrived.
There is no such thing as a product. Products only exist in the minds of the inept. Products only exist for the ‘spoilable’ service they deliver. Services and Service Lifecycle Management is where the check is cashed. Again, it’s much easier for us in aviation to understand these principals since an aircraft is really only a factory for creating available seat miles (ASM) for pax and available ton kilometers (ATK) for freight. Aviation metrics are all denominated in RASM (revenue per available seat mile), CASM (cost per available seat mile), LF (load factor), RPM (revenue passenger mile) and Yield.
Back to our Google Nest example. Even Honeywell Home Automation would probably, privately admit they were asleep at the switch (pun very much intended), they are arguably still asleep. While Honeywell has developed a similar ‘device’ or ‘product’ to Nest, that includes basic remote programming and control of a home’s HVAC, Nest is partnering with electricity and natural gas utilities and heating and air conditioning manufacturers to build a total home energy management offering, servitizing not only their own devices but HVAC’s devices too.
As many airlines have now experienced as they have brought new generation aircraft on board, OEMs are placing onerous constraints on their use of home grown and, or third party technologies. The battle over Operational Data and content has gotten worse. Counter to IT and OT trends in most other industries, with few exceptions, aerospace is becoming more OEM proprietary, non-standard, non-open.
GE invested $105M USD in Pivotal, a provider of cloud IaaS and analytics services. After such an investment, most companies would consider Pivotal a strategic competitive asset and try to lock out competitors. To the contrary, GE has opened their Predix platform to the world. Coopetition at its finest.
Moneyball, changing an unfair game
If you haven’t read Michael Lewis’ book, Moneŷball: The Art of Winning an Unfair Game, or at least seen the movie, I highly recommend it. It’s for anyone who loves baseball or operations research or just a good story about people and family.
Moneŷball examines how Billy Beane, the general manager of the Oakland A’s, used unconventional data and statistics to smartly and affordably assemble a competitive small-market baseball team in the early 2000s. Granted, there is nothing extraordinary about using statistics to win baseball games… or business for that matter. Every kid who collected baseball cards for the past 100 years knows the runs batted in (RBI) or earned run average (ERA) of their favorite players.
Statistician Bill James, a pioneer of sabermetics (the basis for Beane’s Moneŷball approach), took baseball statistical analysis and prediction to the next level when he began publishing books about it in 1970s and 80s. The revolutionary idea that James developed and Beane discovered was that the traditional metrics used by general managers, scouts and teams to forecast player value, for decades, did not actually result in team wins.
For example, instead of ERA, Moneŷball uses Peripheral ERA (PERA), the ‘expected’’ ERA taking into account park adjusted hits, walks, strike outs and home runs. Instead of RBIs, James developed Runs Created (RC) which forecasts the number of runs a hitter is ‘expected’ to contribute to a specific team taking into account the parks that team plays in.
Billy Bean explained the reason for seeking a new way of prediction; “We had to look for new knowledge, we had to question everything. We found great inefficiencies in how everyone was evaluating players. “
Beane broke the biases of his scouting staff, who had always done things their way, by ignoring qualitative opinions like age, appearance, personality and playing style as well as traditional quantitative metrics like batting average and RBIs and instead relied on often overlooked statistics like on base percentage and slugging percentage – numbers that were actually better indicators of a player’s ability to create runs. Because creating more runs than the competition wins games.
As he explained it, “It’s really about information and it’s about making probabilistic decisions – we couldn’t afford to invest something and not get a return. We couldn’t take risk, we had to look at things like an actuary looks at things and understand future decision risk. This is really about getting the most information to make good decisions. We were trying to rob some of the things that were going on in Wall Street and apply them to baseball.”
The strategy worked! The A’s reached the playoffs in 2002 and 2003 and were competitive with the New York Yankees, a team that spent $80+ million more on its roster’s payroll. With this success, the Moneŷball phenomenon transformed baseball…and also the world beyond.
Moneŷball is about reducing risk while increasing the expected value of actionable decisions through quantitative and predictive analysis of a lot of disparate data, more commonly referred to as ‘big data’.
Enter 100 billion sensors, 50 billion connected devices, a yottabyte of data and $14.4 trillion in economic impact.
The consumer and industrial Internet of Things are pontificated to change how businesses deliver everything. IoT is ushering in the biggest and most disparate increase in data seen since the invention of the internet, leading some to say ‘big data’ is the equivalent of ‘oil’ – a currency unto itself.
But you don’t run cars or factories or power plants on oil. Just like oil, big data must be processed into fuel for use; sensor and transactional data needs to be processed and transformed into actionable knowledge before systems and, or people can make decisions and respond in a timely manner.
Accelerating sense and respond and improving accuracy and precision lies at the heart of value creation and value realization in IoT and PdM. Which brings us back to Will Greene; “How do I cash that check?” That simple question anchors every successful CxO’s decision making process. Realizing the value of industrial IoT begins with accurate and precise decision-making focused on the right criteria, outputs and outcomes throughout an organization. That’s the Moneŷball effect. So what does Moneŷball teach us about cashing the check in IoT?
- It teaches us that what is termed the Internet of Things is an equalizer for small to medium sized companies to compete and collaborate with ‘the big guys’;
- That there is sedentary inertia within organizations not to change thus requiring visionary and assertive leadership to overcome the innovator’s dilemma;
- That IoT applies to both planning (buying the right equipment, players, partners) and execution (operations of people, plant, equipment, marketing, sales);
- That merely connecting edge devices does not, in and of itself, realize value;
- That the ability to sense and gather data (big, small, or otherwise) creates potential value that must be realized across the Product and Service Lifecycles in addition to Customer Experience;
- That companies investing in IoT need to look at asset and operations performance levers and how sensing, monitoring, controlling, predicting, diagnosing, prescription, prognosis and autonomics improves decision making to change outcomes; and…
- That predictive analytics (predictive maintenance in industrial markets) delivers the lead-time required to respond to asset, operations and customer events in order to optimize return on assets, return on operations and return on investments.
So, what is the Internet of Things?
The Internet of Things is neither about the Internet nor about Things; it is about creating outcomes (baseball runs and wins or safe on time departure of aircraft).
It’s about connecting edge devices that sense conditions to systems that monitor, analyze and process stochastic events, automatically gathered by both machines and humans, to create value from autonomic manipulation of data into knowledge for the purpose of making timely actionable decisions in order to realize value for consumers of spoilable services.
Contributor’s Details
Michael Wm. Denis
Michael Wm. Denis is a renowned author, speaker and independent consultant providing strategy, business model, organization, marketing, sales, operations and technology advisory services to manufacturers, operators, maintainers and technology vendors focused on optimizing the service lifecycle of complex capital assets. Among other clients, Michael is advising several predictive maintenance solutions vendors’ strategy and business development in the aerospace, aviation and defence markets. www.slm.aero
CaseBank Technologies is a pioneer and leader in delivering diagnostic solutions for complex capital assets that improve first time fix rates, labour productivity and equipment reliability. CaseBank’s Spotlight®, ChronicX® and Diagnostics Data AnalyserTM incorporate actual feedback from field service technicians to accelerate future corrective actions. CaseBank guides the fault isolation processes and procedures to optimize the efficiency and consistency of product support. CaseBank’s solutions are used by maintenance organizations in a variety of industries, including aviation, defence, automotive, rail, industrial equipment, and high tech electronics.
Comments (0)
There are currently no comments about this article.
To post a comment, please login or subscribe.